Listen to this story
|
Historically, running AI/ML workloads on Kubernetes has been challenging due to the substantial CPU/GPU resources these workloads typically demand.
However, things are now changing. The Cloud Native Computing Foundation (CNCF), a nonprofit organisation that promotes the development and adoption of Kubernetes, recently released a new update, Kubernetes 1.31 (Elli).
Register for NVIDIA AI Summit India
Elli introduces enhancements designed to improve resource management and efficiency, making it easier to handle the intensive requirements of AI and ML applications on Kubernetes.
Enterprises are increasingly turning to cloud native applications, especially Kubernetes, to manage their AI workload. According to a recent Pure Storage survey of companies with 500 employees and more, 54% said they were already running AI/ML workloads on Kubernetes.
Around 72% said they run databases on Kubernetes and 67% ran analytics. Interestingly, the numbers are expected to rise as more and more enterprises turn to Kubernetes. This is because the development of AI and ML models is inherently iterative and experimental.
“Data scientists continually tweak and refine models based on the evolving training data and changing parameters. This frequent modification makes container environments particularly well-suited for handling the dynamic nature of these models,” Murli Thirumale, GM (cloud-native business unit), Portworx at Pure Storage, told AIM.
Kubernetes in the Generative AI Era
Kubernetes marked its 10th anniversary in June this year. What started with Google’s internal container management system Borg, has now become the industry standard for container orchestration, adopted by enterprises of all sizes.
The containerised approach provides the flexibility and scalability needed to manage AI workloads.
“The concept behind a container is to encapsulate an application in its own isolated environment, allowing for rapid changes and ensuring consistent execution. As long as it operates within a Linux environment, the container guarantees that the application will run reliably,” Thirumale said.
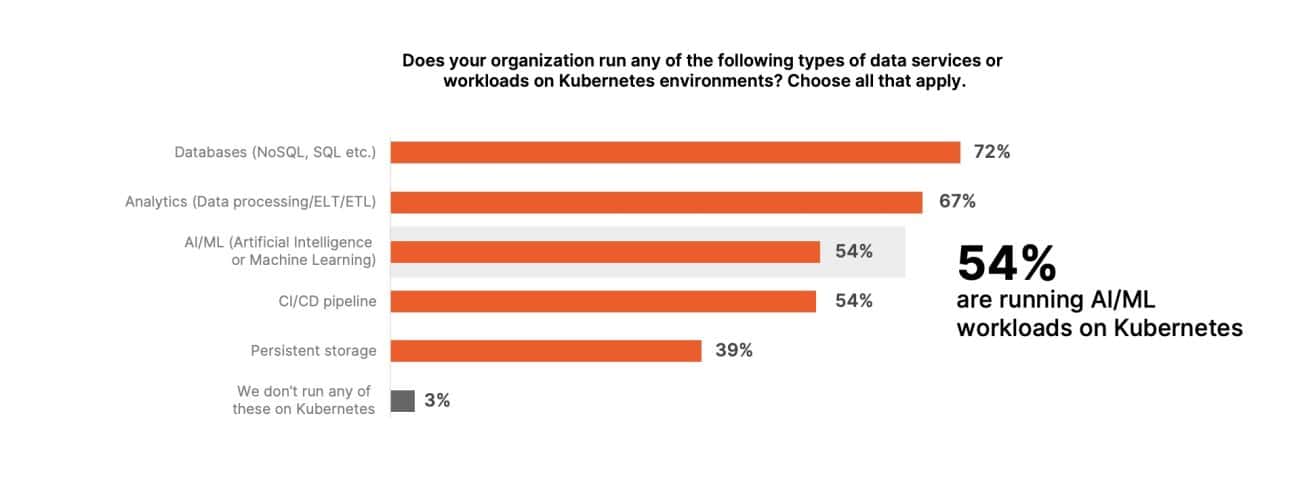
(Source: The Voice of Kubernetes Expert Report 2024)
Another reason AI/ML models rely on containers and Kubernetes is the variability in data volume and user load. During training, there is often a large amount of data, while during inferencing, the data volume can be much smaller.
“Kubernetes addresses these issues by offering elasticity, allowing it to dynamically adjust resources based on demand. This flexibility is inherent to Kubernetes, which manages a scalable and self-service infrastructure, making it well-suited for the fluctuating needs of AI and ML applications,” Thirumale said.
NVIDIA, which became the world’s most valuable company for a brief period, recently acquired Run.ai, a Kubernetes-based workload management and orchestration software provider.
As NVIDIA’s AI deployments become more complex, with workloads distributed across cloud, edge, and on-premises data centres, effective management and orchestration get increasingly crucial.
NVIDIA’s acquisition also signifies the growing use of Kubernetes, highlighting the need for robust orchestration tools to handle the complexities of distributed AI environments across various infrastructure setups.
Databases Can Run on Kubernetes
Meanwhile, databases are also poised to play an important role as enterprises look to scale AI. Industry experts AIM has spoken to have highlighted that databases will be central in building generative AI agents or other generative AI use cases.
As of now, only a handful of companies are training AI models. Most of the remaining enterprises in the world will be finetuning their own models and will look to scale with their AI solutions very soon. Hence, databases that can scale and provide real-time performance will play a crucial role.
“AI/ML heavily rely on databases, and currently, 54% of these systems are run on Kubernetes—a figure expected to grow. Most mission-critical applications involve data, such as CRM systems where data is read but not frequently changed, versus dynamic applications, like ATMs that require real-time data updates.
“Since AI, ML, and analytics are data-intensive, Kubernetes is becoming increasingly integral in managing these applications effectively,” Thirumale said.
Replacement for VMware
Broadcom’s acquisition of VMware last year also impacted the growing usage of Kubernetes. The acquisition has left customers worried about the pricing and integration with Broadcom.
“It’s a bundle, so you’re forced to buy stuff you may not intend to,” Thirumale said.
Referring to the survey again, he pointed out that as a result around 58% of organisations which participated in the survey plan to migrate some of their VM workloads to Kubernetes. And around 65% of them plan to migrate VM workloads within the next two years.
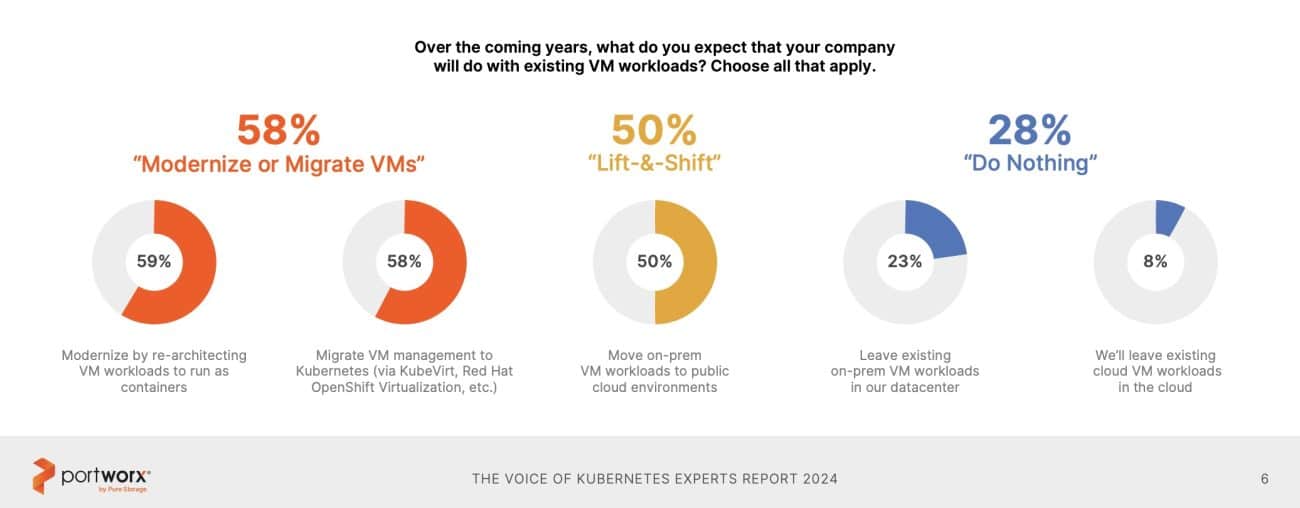
Kubernetes Talent
As enterprises adopt Kubernetes, the demand for engineers who excel in the technology is also going to increase, and this will be a big challenge for enterprises, according to Thirumale.
“Kubernetes is not something you are taught in your college. All the learning happens on the job,” he said. “The good news is senior IT managers view Kubernetes and platform engineering as a promotion. So let’s say you’re a VMware admin, storage admin, if you learn Kubernetes and containers, they view you as being a higher-grade person,” he said.
When asked if education institutions in India should start teaching students Kubernetes, he was not completely on board. He believes some basics can be taught as part of the curriculum but there are so many technologies in the world.
“Specialisation happens in the industry; basic grounding happens in the institutions. There are also specialised courses and certification programmes that one can learn beyond one’s college curriculum,” he concluded.