Listen to this story
|
For the first time in this century, TIME magazine released a list dedicated to 100 most influential personalities in AI. Yet, amidst the grand spectacle, some important individuals in AI found themselves absent from this illustrious list.
In continuation with the Part-1 that we published earlier, here’s a list of all the polymaths who couldn’t make it to TIME.
Erik Brynjolfsson
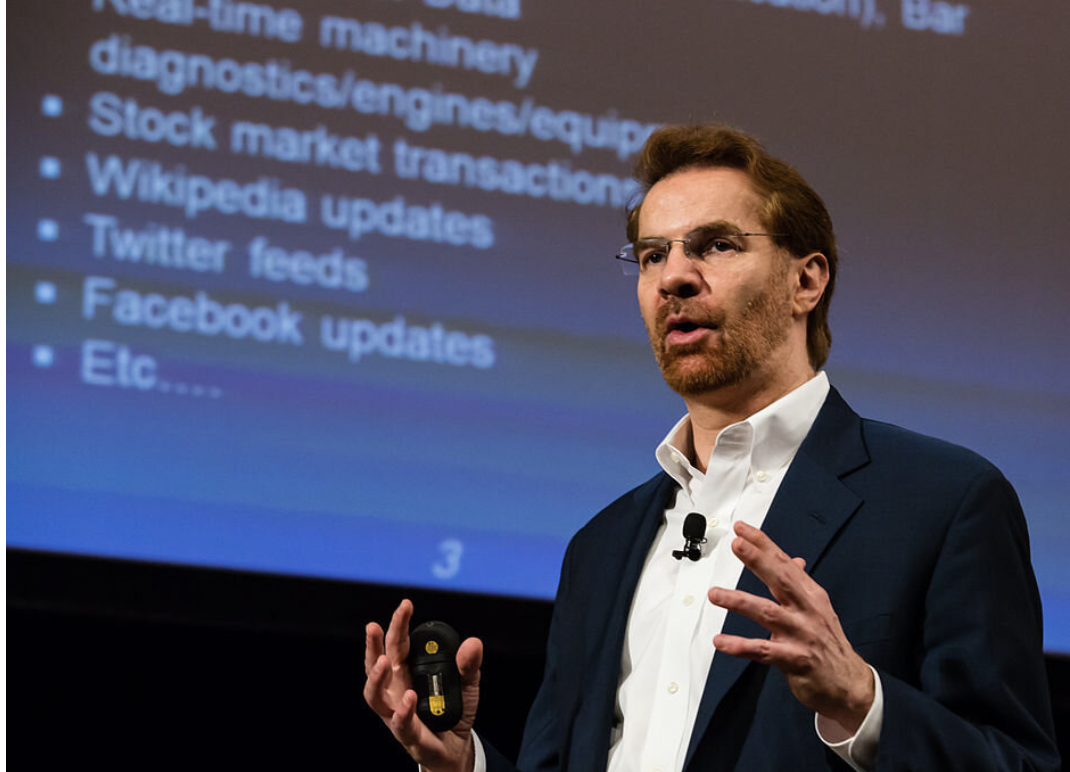
Erik Brynjolfsson, currently a professor and a senior fellow at Stanford University, is a visionary scholar and guiding star in digital economics. He has been a driving force in the study of the digital economy, emphasising the profound transformation brought about by technological advancements. While the rise of AI sparks concerns about job displacement, Brynjolfsson offers a more pragmatic perspective. In an NYT piece, Brynjolfsson encourages us to shift our gaze. “The thing that I wish people would do more of is think about what new things could be done now that were never done before. Obviously, that’s a much harder question,” he said. It is also, he added, “where most of the value is”.
Rodney Brooks

Then there’s Rodney Brooks, a seasoned technologist who knows the difference between real progress and baseless hype as a majority of his predictions have been spot-on. Having co-founded iRobot and contributed significantly to MIT’s computer and AI labs, his expertise in robotics and AI is unparalleled. Brooks, in his annual predictions, reminds us to temper our expectations, believing that the integration of robots into our lives will be a gradual, symbiotic process.
In his fifth annual scorecard in 2023, he confessed to having allowed hype to make him too optimistic about some developments. “My current belief is that things will go, overall, even slower than I thought five years ago,” he wrote.
Brooks expects “robots that will roam our homes and workplaces … to emerge gradually and symbiotically with our society” even as “a wide range of advanced sensory devices and prosthetics” emerge to enhance and augment our own bodies: “As our machines become more like us, we will become more like them. And I’m an optimist. I believe we will all get along.”
Yejin Choi

Despite AI breakthroughs in previously human-dominated language and visual art, our gravest concerns should probably be tempered, believes Yejin Choi, a professor of computer science at the University of Washington. The computer scientist, who is also a 2022 recipient of the MacArthur “genius” grant, has been doing groundbreaking research on developing common sense and ethical reasoning in AI.
She reminds us that simply instructing AI not to commit certain actions is insufficient; AI must also possess the wisdom to make sensible decisions and consider the broader implications of its actions. In an interview with the NYT earlier this year, she elaborated how some people naïvely think that if we teach AI “don’t kill people while maximising paper-clip production”, that will take care of it. But the machine might then kill all the plants. It’s common sense not to go with extreme, degenerative solutions, she explained.
Jeff Dean

American computer scientist and engineer Jeff Dean has long headed the AI department at Google Brain, encouraging researchers to publish academic papers actively. Impressively, they officially pushed out nearly 500 studies since 2019, according to Google Research’s website.
On the one hand, there are concerns around AI development and its associated risks. And on the other, this is a natural progress in technology: Innovation happens quickly. It’s not an either/or; it’s a both/and. To Dean’s point, society can mitigate risk and be bold. Time and again, Dean has reminded us that the rapid development of AI is both exhilarating and worrisome, emphasising the need to balance innovation and risk mitigation.
Sergey Levine
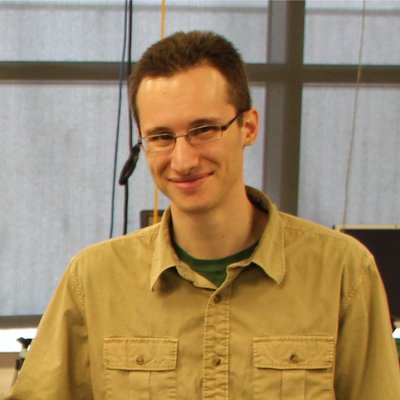
Levine is the associate professor of electrical engineering and computer sciences and the leader of the Robotic AI & Learning (RAIL) Lab at UC Berkeley. An advocate of reinforcement learning who also holds an appointment with the Robotics at Google program, along with fellow researchers Julian Ibarz, Jie Tan, Chelsea Finn, Mrinal Kalakrishnan, and Peter Pastor, recently published a review titled How to Train Your Robot with Deep Reinforcement Learning — Lessons We’ve Learned.
In the latest, second of four Distinguished Lectures on the Status and Future of AI that he has delivered, he extensively spoke about examining algorithmic advances that can help ML systems retain both discernment and flexibility.
He emphasised the relationship between data and optimisation in problem-solving. Without adequate data, researchers are unable to address challenges innovatively. Conversely, optimisation strategies struggle to find real-world applications without the necessary data. By combining both the elements effectively, we can inch closer to creating a space-exploring robot capable of devising solutions to unexpected problems, Levine believes.
Pieter Abbeel
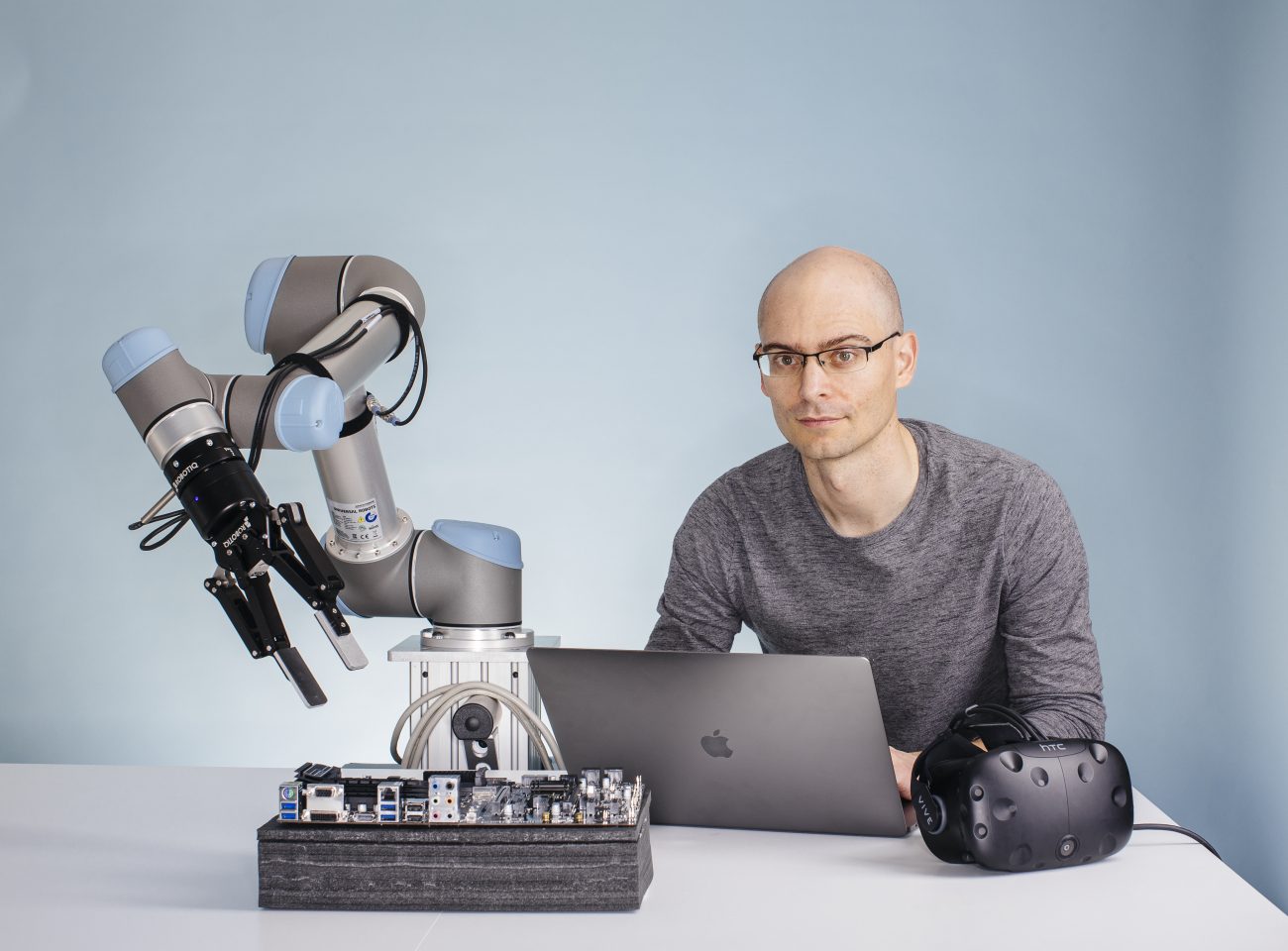
Peter Abbeel has had a long and upward career in robotics from learning to significantly improve robot manipulation to receiving the 2021 ACM Prize in Computing for pioneering work in robot learning. Abbeel has journeyed from teaching robots to learn from humans to pioneering learning-through-trial-and-error techniques. His groundbreaking work forms the bedrock of the next generation of robotics, showcasing the potential of AI to evolve and adapt.
He is currently a professor of electrical engineering and computer sciences, director of the Berkeley Robot Learning Lab, and co-director of the Berkeley AI Research Lab at the University of California.